Research News
Large Language Model Accurately Predicts Online Chat Derailments
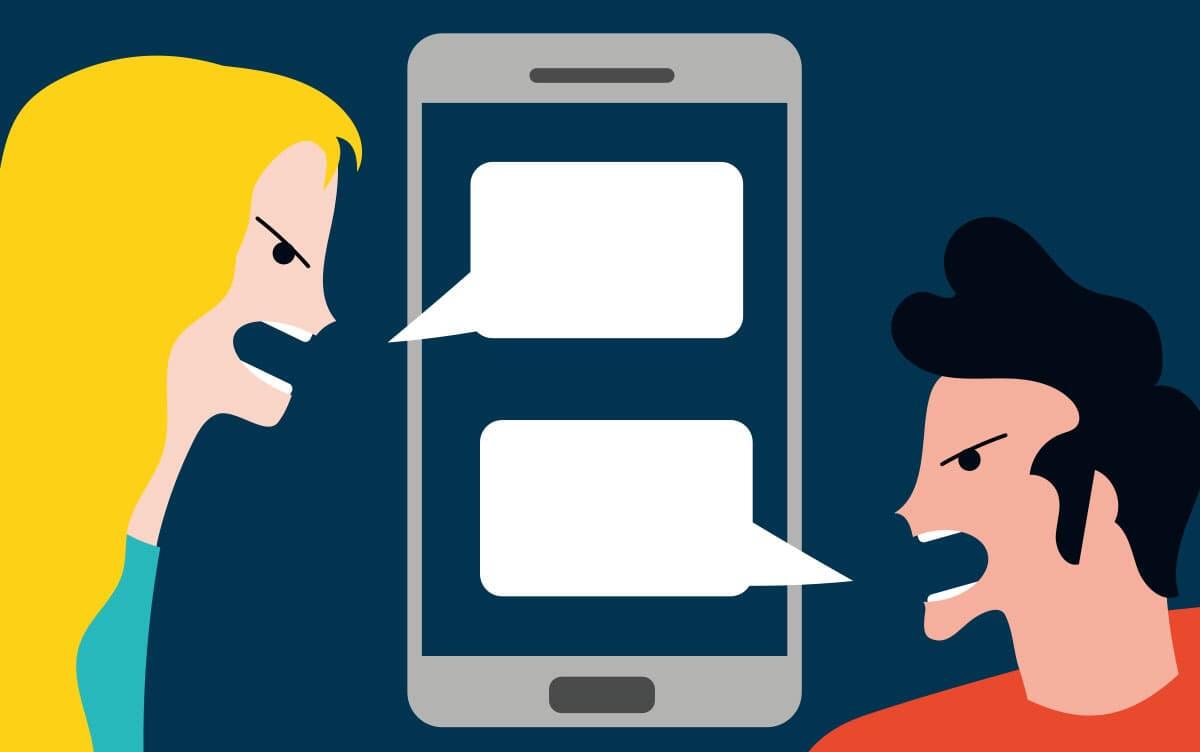
Researchers at University of Tsukuba have developed a method to predict when online conversations deviate from their original topics and escalate into personal attacks. Using large language models (LLMs) and a zero-shot prediction approach, the technique achieves high accuracy without requiring platform-specific training.
Tsukuba, Japan—Online chat rooms and social networking platforms frequently experience harmful behavior as discussions drift from their intended topics toward personal conflict. Traditional predictive models typically depend on platform-specific data, limiting their applicability and increasing implementation costs.
In this study, the researchers applied a zero-shot prediction method to LLMs to detect conversational derailments. The performance of various untrained LLMs was compared to that of a deep learning model trained on curated datasets. The results showed that untrained LLMs achieved comparable, and in some cases superior, accuracy.
These findings suggest that platform operators can implement effective moderation tools at reduced cost by leveraging general-purpose LLMs, supporting healthier online communities across diverse platforms.
Original Paper
- Title of original paper:
- Zero-Shot Prediction of Conversational Derailment With Large Language Models
- Journal:
- IEEE Access
- DOI:
- 10.1109/ACCESS.2025.3554548
Correspondence
Associate Professor YOSHIDA Mitsuio
Institute of Human Sciences, University of Tsukuba
NONAKA Kenya
Doctoral Program in Risk and Resilience Engineering, Degree Programs in Systems and Information Engineering, University of Tsukuba
Related Link
Institute of Business Sciences
Master's / Doctoral Program in Risk and Resilience Engineering